Quant and qual. A marriage to bring focus to the “what”, the “where” and the “why”
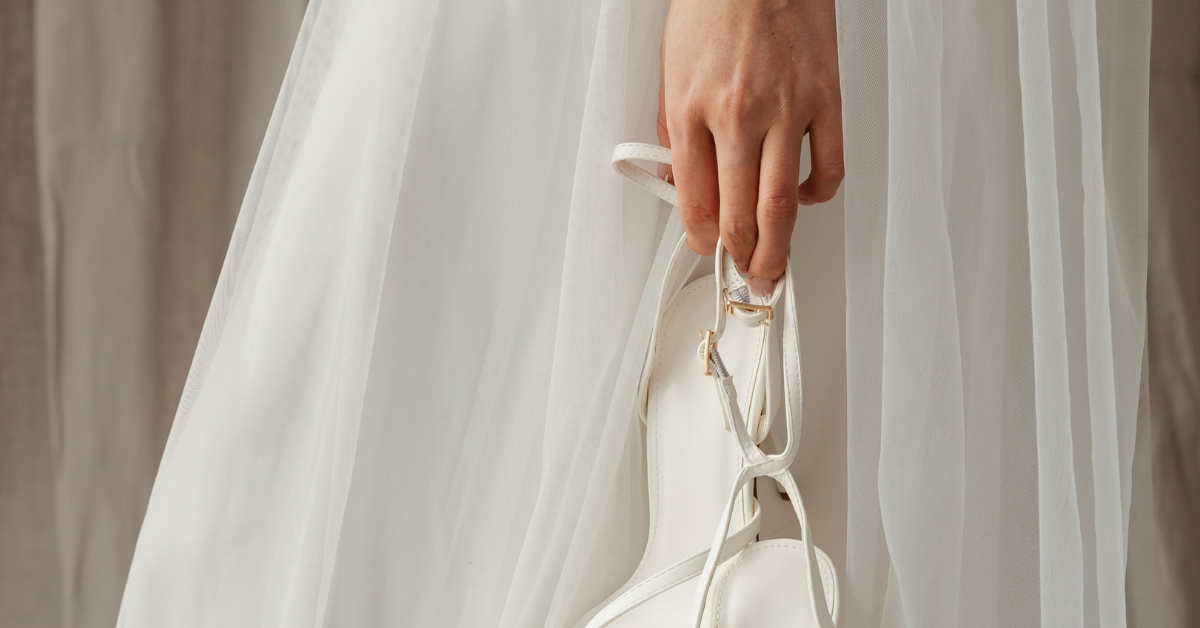
Welcome to this archived 5-Minute-Friday Newsletter. If you’d like to subscribe to be the first to read these and have them hit your inbox each Friday, subscribe below.
Start.
I usually kick off each newsletter with a short summary of what to expect in reading on, but this week I knew I could sum it up in one meme.

In today’s highly digital world, we often have no shortage of quantitive data at our disposal. Today’s discussion explores why sometimes, this is exactly the problem.
We often treat each type of analysis distinctly, though it’s in aligning insights from both we often arrive at a much more informed hypothesis for testing.
The role of quant
If you’re subscribed to this newsletter, I don’t think I really need to give any explanation for the role quantitive analysis plays in our ability to bring forward clarity and focus to our initiatives. To be honest, it’s probably the people who wouldn’t subscribe to this newsletter (the “shoot from the hip only folk”) who need it most, so I won’t labour on this point.
Suffice to say, quantitive analysis can bring forward clarity of the “what” and the “where”. That is, “what should we be looking at?” and “where specifically should we be looking?”
Since I know I have some B2B marketers in the crowd, let me offer a B2B example. If you have any kind of form on your site, you can apply the same example. Or if you’re an online retailer, you could simply replace the concept of “form fallout” for a “checkout fallout analysis”. Same concept.
Take the promotion of an online webinar.
300 people visited the landing page.
Of these 300, 80 bounced, and 220 scrolled to a depth of 60% reaching the form at the bottom of the lead page.
Of the 220 who arrived at the form, 200 engaged with the form in some way (that is to say, they clicked the first form field).
… yet only 100 people actually submitted the form.
So, why didn’t they complete the form?
This is the question that is often asked – and it IS the question we want answered but this is exactly what confuses us in thinking quant alone will tell us the answer to that question of “why”.
It actually only helps us refine “where” we should keep looking. What a sick trick.
A clever analyst will exhaust the quant they have at their disposal to be very specific about “where” we should look, asking themselves questions like…
How many of the users were new vs returning?
What were their persona or affinity categories? Their location? Their propensity to be interested in the topic?
Are there any particular device types or browsers that see more non-submits than successful submissions?
The best case scenario is that we find a significant volume and a clear trend that isolates one hypothesis for testing, yet sometimes there simply isn’t one.
The insight is, there is no clear insight.
It’s at this time I often observe many decision makers continue to point to the analyst requesting continuous (sometimes never-ending) digging in the quant quarry of data in the hope they’ll find that clarity via a little nugget of golden insight.

The result of doing this is often continuous testing and small, iterative lifts to conversion. Don’t get me wrong, I’m all for embedding a process of data-driven experimentation but when there is no clear hypothesis and results are stagnating, it’s time to consider whether there is a way to speed things up and gain further clarity around our customer needs…
This is your call to action to consider that balance of when to keep digging, inching towards improvements over time – and when to check that you’re not missing something by limiting yourself to the data you have now.
So how do we get clarity on the “why”? Enter our friend qual…
The result of doing this is often continuous testing and small, iterative lifts to conversion. Don’t get me wrong, I’m all for embedding a process of data-driven experimentation but when there is no clear hypothesis and results are stagnating, it’s time to consider whether there is a way to speed things up and gain further clarity around our customer needs…
This is your call to action to consider that balance of when to keep digging, inching towards improvements over time – and when to check that you’re not missing something by limiting yourself to the data you have now.
So how do we get clarity on the “why”? Enter our friend qual…
The role of qual
One analogy I’ve loved was shared by Jenni Bruckman in an Analytics Power Hour podcast. I’m adding a little extra colour to the analogy but the sentiment is the same.
Consider you might be looking to purchase an investment property with a budget set aside to renovate and give the home a little facelift. Quant first helps us narrow down what house to buy in what street. What do we think will likely generate us the best return on our investment? The “what” might be a three bedroom home and the “where” might be nearby a local school in a sought after location. We’ve gotten so far as choosing a home suitable for a growing family, ensuring it meets some foundational family needs. Check.
So the quant focused our initial direction. Even still, how do we consider what style will appeal to others and maintain a high resale value? If you have an eclectic taste and choose to renovate using a very unique set of colours and textures, you might find yourself whittling down your market quite substantially. The reason one might choose your home vs another can be just as much emotional as rational. It might tick every box on their list but yellow just isn’t their colour. “Why”?, Well you’d have to ask them…

Just as Jenni shared on Analytics Power Hour podcast, “The quant can tell you where to place the ladder but it can’t tell you what colour to paint the house”.
“So… what colour do I paint the house, Kate?”
Time to gather some qual data and tap into that “Voice of Customer”.

But enough of this house, let’s go back to our practical example from earlier.
In the context of asking ourselves “why” we’re seeing a 50% fallout rate from people who start vs start and complete the form.
- Are the “required” fields on the form requesting data customers may not feel comfortable sharing? OR is there an error on the form displaying for some browsers?
- Is there simply too much friction presenting a barrier to complete? Are our busy customers getting distracted before being able to submit all of the questions we’ve requested answers for? Is all the information we are requesting of key importance?
- Are prospects requiring more information on the time of the webinar? Such as knowing a recording will be shared if they’re unable to attend? Or allowing them to conduct a time conversion?
Or the kicker…
- Are we talking to the right group of people and serving them the right content for their needs?
As consumers, we often have the ability to put ourselves in the shoes of our prospects to further form some additional hypotheses for testing.
These hypotheses can be useful to keep in mind when conducting qualitative research whether it be observational or in surveying / interviewing customers. Though be careful not to fall into the trap of asking “leading” questions.
Statistically, we want to construct qualitative analysis with the rigour required to ensure significance in the results we seek and ideally, your analyst is best placed to support with that construction. We also want this effort to plug gaps and ideally, can be stitched to some of the data available quantitatively (providing privacy policies permit) for more rigorous segmentation and analysis – so be sure to get your analytics team involved.
A marriage made in heaven
If nothing else, I hope you leave today’s read with the prompt to consider exactly the question you are asking and what data you are looking at to answer it.
Quant alone can and often does fuel an incredible backlog for continuous experimentation isolating the “what” of the problem (and its opportunity size) and the “where” to focus.
Qual alone can bring an incredible perspective and focus for where to start your quantitative analysis and refine your value proposition, bringing forward “why” customers want what they want.
Absolutely continue to use them individually – though if you find yourself spotting these signs, it’s time to start using them together.
- You’re feeling like you are trying to get blood out of a stone from your quantitive data
- You’re feeling like you are making a very broad hypothesis for “why” with little quantitive volumes“MAYBE customers are thinking like this….”
- You’re feeling like you’ve been experimenting for some time but still see stagnant results
Take these as signs to revisit your “what”, “where” and “why”. Sometimes that means getting creative and going back to collecting more data.
End.
Leave a Reply