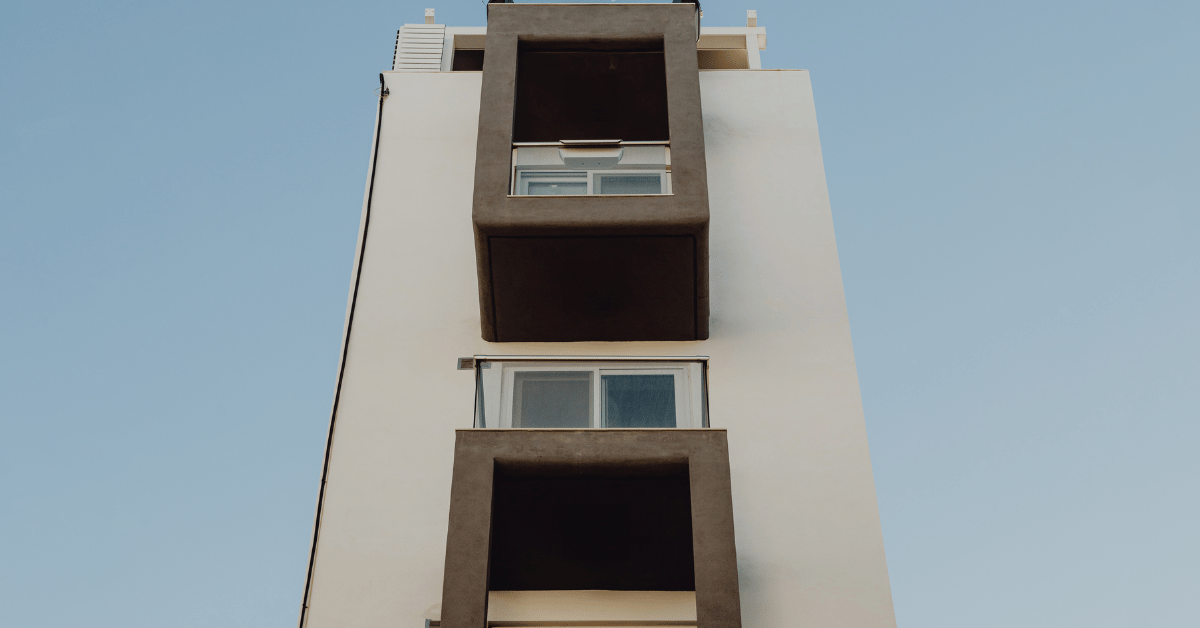
My good friend Robyn is back.
I’ve had not one but two requests about whether we still whip up Robyn Media Mix Modelling code outputs or I like to call them, MMM “Proof of Concepts” (POC’s) at The Lumery and the answer is yes, we do.
I suppose despite all the noise I was making about all things MMM a year ago, businesses are finally cottoning on to the fact that this will truly only be the way to solve for the losing visibility we are faced with due to the depreciation of cookies. At least with the level of visibility and sample sizes that can make recommendations meaningful.
I always say, all data is sampled and as mentioned on my podcast with Jim Gianoglio on Measure Up recently, I’m not yet seeing such dire changes in our MTA models (yet) but perhaps some are. Or perhaps people are finally preparing ahead of time as they should be (just like so many didn’t do, and still haven’t done for GA4).
A lot has progressed in the land of Robyn since we ran our first version of it, while the version we know now was still in beta and the first model took 6 hours to run…. Wow they’ve come a long way. They also seem to have a fancy new logo now too. Same colours though…

If you had previously come to know that logo on the left, remove it from your memory. Facebook I mean Meta’s Robyn has had a rebrand
Even still, the core concepts of all MMM, including Robyn remain the same so I thought this week would be a good opportunity to recap what a Marketing/Media Mix Model is, why you need one and specifically, what you can get out of Meta’s freebie open source code version Robyn.
Above and beyond Robyn there are actually many alternatives. There’s “Build from Scratch”, “Buy” and then what we like to call, “Build to Buy”.
It would require a whole other newsletter to dig into all of the detail within each of the above options but at a glance, here’s what you need to know about your options in the MMM landscape.
If this topic interests you and you want a deeper look into Build, Buy and Build to Buy Open Source MMM options, hit “reply” let me know.
Option A: Build from scratch
Build from Scratch is bloody expensive (~$200 – ~$500K), timely, requires you to have some phenomenal data scientists. You’ll always have bias in any model but arguably, some more in this approach since you’re pinning the influences on few individuals (as opposed to a “buy” option below that would be informed and calibrated by multiple businesses). BUT if you can handle all of that, “building from scratch” will offer you all the flexibility in the world.
Option B: Buy
Buy is also, not hugely cheap but it’s cheaper than hiring or paying a highly skilled data scientist, and because it’s a product, off the shelf, you know what you are getting, loosely how it works and have the support services you need like any other platform you buy. I’m a big fan of the work the team at Mutinex are doing (I’m meeting with them today, actually) and have followed along with the intelligent noises of Michael Kaminskey of Recast in the US too. Like any product, you have to have a conversation to get exact pricing but for most MMM products you’re looking at ~$120 – $200K.
Option C: Build to Buy
Build to buy is a term our team uses for the very specific use case of marketers who are dying to get their hands on an MMM but who struggle to unlock the budget for a long term contract or bespoke build.
In ~6 weeks (and around ~$50 – $80K) any clever data scientist can standardise and transform your data for import, and then rip an open source code from either Meta’s Robyn or Google’s Lightweight MMM to whip up your very first POC. For clients of ours who are really looking to demonstrate the value that can be derived from marketing analytics, this is a great ‘quick start’ option. If you’re clever enough to do all the data standardisation yourself and you know what variables you need to include (there’s a bit of an art to crafting an MMM strategy) there is also a DIY tool on the market called Cassandra where a clever MMM specialist called Gabrielle Franco built an interface to sit on top of Facebook’s Robyn.

So which one is right for you?
Whether you have the budget to go all-in or you are dipping your toes in the water with your first MMM, there’s a lot to consider. Among them is how you will communicate the output and encourage your teams to understand and adopt the model results for decision making.
You can get lost in the world of the details within all of the options above. Ultimately George E. P. Box said it best.
Regardless of what option you do choose, the output, while offered at varying levels of translation, largely provides you with the same set of statistics to make decisions.
An MMM will give you the following:
- Definitive channel ROI: We know the funnel isn’t linear and there is a bunch of overlap. Linear regression is one way we help solve for that complexity and arrive at a single, definitive number from which to make decisions.
- Point of diminishing returns for each channel: How much life do you have per channel? If you were to continue popping in a new dollar to each channel, at what point would you reach your peak of making money vs starting to reduce your ROI? What’s your tipping point?
- Contribution of key impacts: Is your business seasonal, really? How many sales do you generate simply because it’s Summer or Christmas vs what your spend realistically supported you to drive?
- Channel ad/decay rate: How much life do some channels have over others? Is a TV impression worth the same as a radio one? What about as a viral TikTok content piece? Unpick content and message effectiveness using this metric.
Want to see more where this came from (and a bit of a view of what the Robyn MMM Output looks like) check this throwback to V1 of Attribution Gal branding on LinkedIn here.

Old branding or new branding? What do you reckon?
It’s a good reminder to keep in mind that regardless of where you start your MMM journey, over time you will no doubt want more granularity, more streamlined and automated data ingestion, more frequent output and a tailored UI to play with the inputs and outputs from the model.
But matching maturity for where it makes sense to start is important. Biting off more than you can chew and is realistic to maintain may cause paralysis and confusion, so if you’re not sure where to start, start with a POC and build from there.
Even with an imperfect MMM, the output can still provide a ~15 – 20% return on spend. I was overjoyed to work with an Australian beauty brand to model an uplift of 23% through both reallocation, and a slight bump in investment to some channels that were yet to see the point of diminishing returns. So you can in all likelihood see it pay for itself if you have a sizeable media budget.
Want to learn more about MMM? Hit reply and let me know what you are interested in and I’ll continue the analysis in next week’s newsletter. Until then.
Leave a Reply