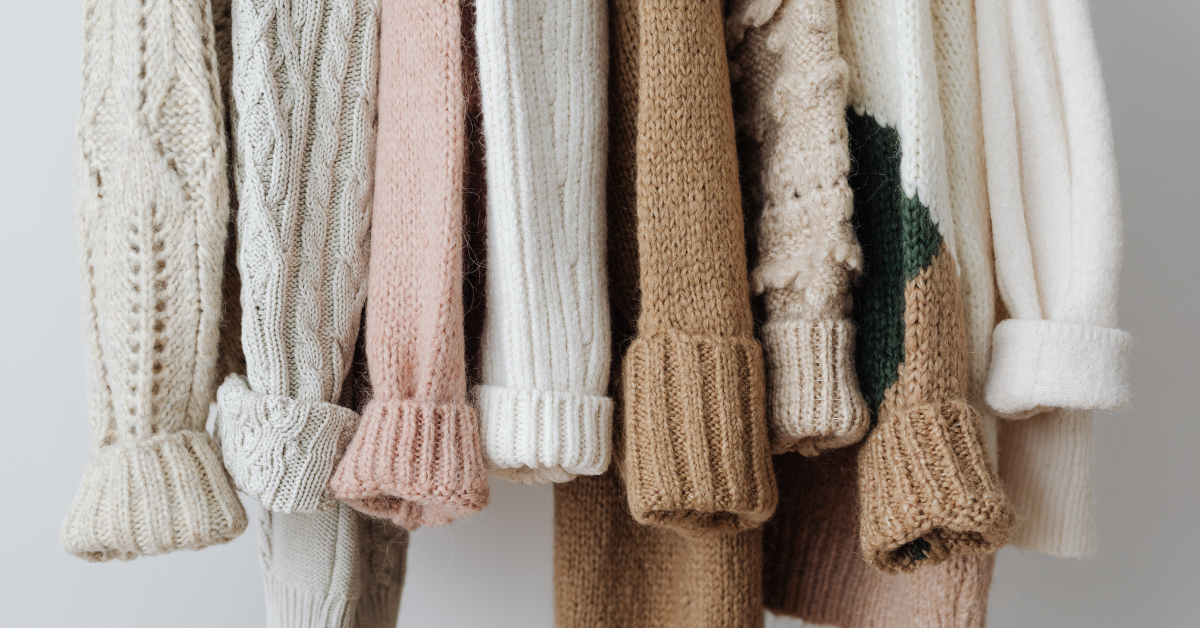
This week I had the opportunity to work closely with one of my team members to untangle a web of metrics to put forward a clear, concise narrative and set of recommendations.
Basically the absolute favourite part of my job.
Translating business needs back to analytics professionals to dig deeper into data, then translating back into business speak, all while mentoring the next generation? Yep, dream job.
Our task this week was “just another experimentation report” in many ways, but one where we had an opportunity to explore an additional data set to layer in new perspectives, then, understand whether we had more or less signals directing us to the next hypothesis.
Spoiler: we had more.

The client we were supporting recently onboarded Contentsquare. For those not familiar, Contentsquare is a digital experience intelligence and analytics platform. It offers deep, qualitative snapshots while layering quantitative analytics data into reports to better understand how customers are navigating through a site.
Review direct user recordings, check for key hygiene issues and bugs (such as rage clicks as per this monkey’s experience), easily detect trending page journeys, understand form and checkout engagement by field and more.

Contentsquare sits in the same family as tools like HotJar, Mouseflow and other heatmapping software but layers in a lot of quantitative metrics direct within the platform for ease of combining the “what outcome happened” with the “why we think users behaved this way”.
This week we were looking at transaction types used on checkout.
Could we improve the way these were laid out on the page both to support the user experience in navigating what makes sense to them, while making the businesses preferred method more prominent on the page?
We looked at our Google Analytics (GA) data to detail the transaction counts. While some achieved statistical significance, many didn’t. This doesn’t tell us much.
As my team member and I say to one another: “No sig, no play”.

No statistical significance merely tells us that the data we are seeing could be due to random chance. The signal is not strong enough to derive insight from. I know it can be hard to wrap your head around, but it’s important to ignore this. The only exception is if the potential risk and opportunity cost is significantly high and the business is not willing to take on that risk in the pursuit of a statistically quantified finding (i.e. if revenue is falling through the floor). At that point though, it’s still important to remember it’s not that the test failed, but rather that the risk of gaining the learnings exceeded the risk threshold of the business, so winding back the test to baseline is the only suitable path forward.
But I digress. We had some strong signals in the GA data but only few.
So, we looked at Contentsquare.
It’s important to note that Contentsquare gathers its data by dropping a simple tag on the website and effectively “scraping” events from the site code. Though I don’t have all of the technical details of the implementation, I suspect having a well constructed data layer will only serve to provide greater consistency across all MarTech platforms.
Even still, unless MarTech platforms are implemented with best practice data collection standards in mind (that is, ensuring all events are collected in a single, server side location and sent to any and all platforms as needed) the data itself is likely to have discrepancies across platforms.
Here’s where our handy cognitive dissonance comes into play

I’ve spoken before about analytics often being about needing to hold two truths, that sometimes conflict, in our minds at one time.
“Revenue data in Google Analytics says $XXX,XXX”
“Revenue data in Contentsquare says $XXX,XXX”
Here’s what NOT to ask:
“Which is right!? How can we trust these insights unless they are consistent and aligned to our ACTUAL transaction data?”
Sure, there’s a level of confidence we need that the implementation is capturing a representative sample, but I’ve said it before and I’ll say it again. Everything is sampled. Except maybe for your actual bank and transaction data… but in the digital world, consider your insights, sampled.
We don’t need to be distracted chasing exact match data across platforms. It’s better if they are, even if just to help avoid the above distraction (losing trust in data is the fastest way to lose momentum and buy-in towards data-driven decisioning) and as an aside, effective MarTech data layer implementations will solve this problem…
Until then though, what we need to be more concerned about is that DIRECTIONALLY the data tells the same story.
Ours did.

Beyond this, our Contentsquare data, which offered the actual revenue figures achieved significance, so we had a data set we could use to pinpoint what was happening as a result of the test.
Layering insights from both platforms gave us our story.
We compared and contrasted where both data sets aligned and the few cases where they conflicted (yet, with one data set not having achieved significance, so an unreliable conflict that could be removed from consideration).
Digging deeper we were able to see how the experiment variation influenced an entirely different set of behaviour for how users engaged with transaction types. As it turned out, the transactions the design team and choice of language used in the redesign actually drew further attention to the transaction types that were intended to be “deprioritised”.
Go figure.
This is why we test.

So a few little important reminders in this week’s experience I’ll leave with you.
- Investigate leveraging multiple data sets to expedite learnings (and get more of the qualitative “why” as well as the quantitative “what”)
- So long as your implementation has been QA’d with a level of confidence you can stand behind, your directional insights matter more than scrutinising and cross checking every data point across platforms.
- Don’t allow yourself to be distracted by results that did not achieve significance – a conflict is only a conflict if both sets of results are significant and they are telling you different things.
Leave a Reply